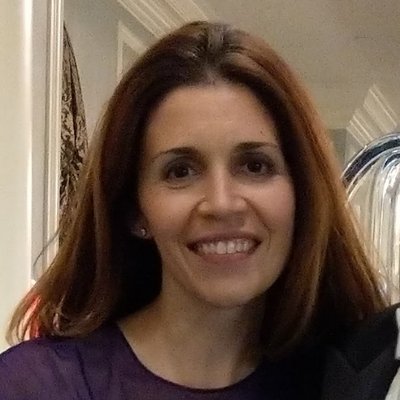
Christine McLeavey
Christine is a Member of the Technical Staff at OpenAI, where she recently published Jukebox and MuseNet. She studied physics at Princeton, and neuroscience and medicine at Stanford. Also a trained classical pianist, she then moved away from science to work as a musician. This blog is mainly from the summer of 2018, and follows her path studying deep learning and reinforcement learning, as well as her project during the OpenAI Scholars Program. Please follow her on Twitter for updates.